Quantum computing is a revolutionary technology that harnesses the principles of quantum mechanics to solve complex problems far beyond the reach of classical computers. Unlike traditional systems that process information in binary bits (0s and 1s), quantum systems leverage quantum bits, or qubits, which can exist in multiple states simultaneously. This ability enables quantum computers to perform calculations at unprecedented speeds, offering a quantum advantage that could transform industries such as healthcare, finance, and logistics.
The potential of quantum computing lies in its ability to tackle problems that are too complex for classical methods. For example, quantum computing research has shown how quantum systems can be applied to simulate quantum processes, aiding in everything from drug discovery to material science. These real-world applications are already making waves, with companies like Google Quantum AI leading the way in the development of quantum algorithms.
In fields such as AI and machine learning, quantum computing is enhancing data processing and speeding up tasks that require error-corrected models. In finance, it is revolutionizing financial modeling and risk analysis by quickly evaluating complex variables. Meanwhile, in traffic optimization, quantum computing is showing promise in managing real-time systems for smarter cities.
As quantum computing evolves, its practical applications will continue to grow, making it a game-changer for solving some of the world's most pressing challenges.
What is Quantum Computing?
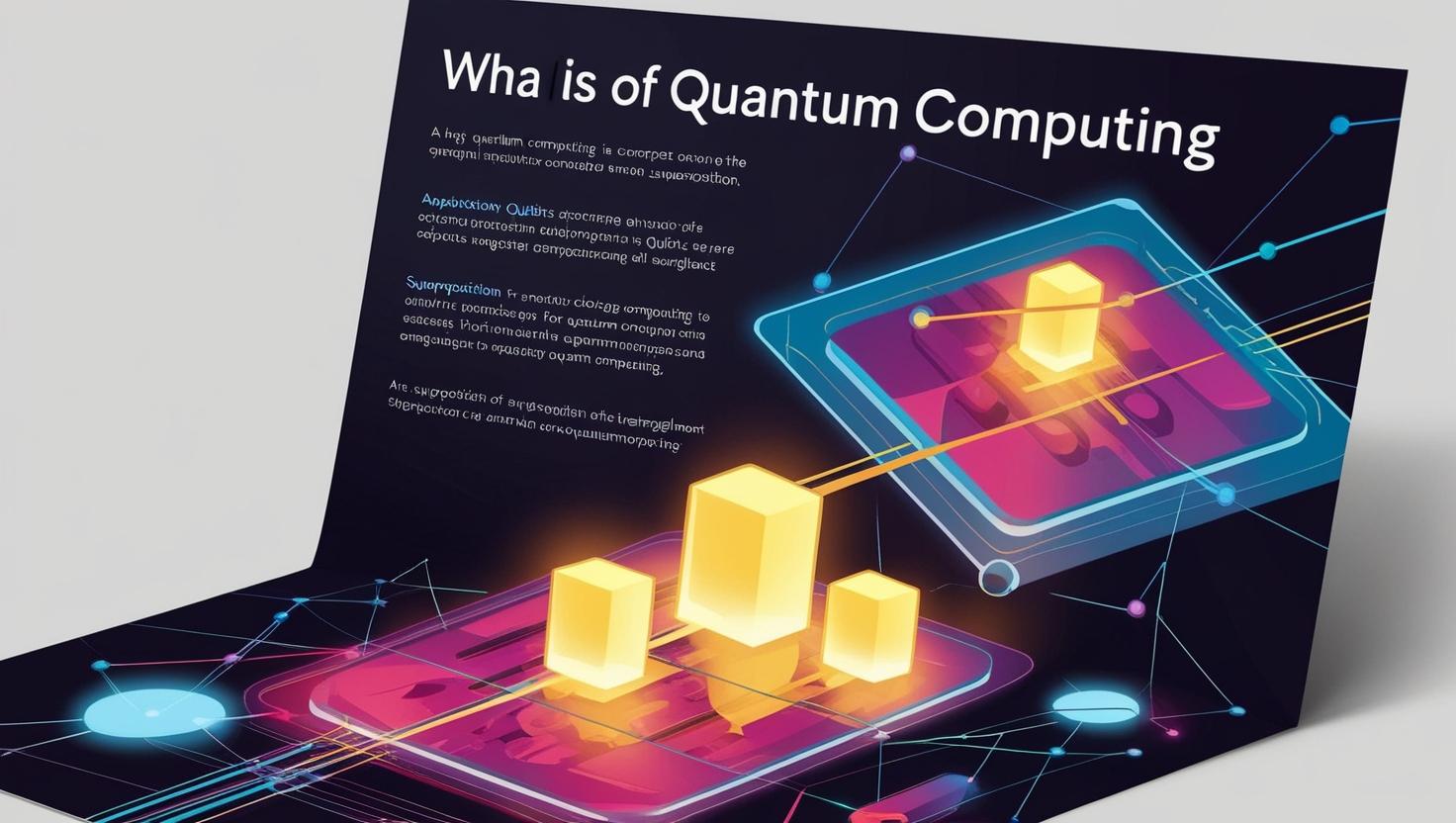
Quantum computing is an advanced field of technology that leverages the principles of quantum mechanics to process information in ways that classical computers cannot. At its core, quantum computing uses quantum systems to perform calculations, tapping into quantum phenomena such as superposition, entanglement, and quantum interference. These concepts allow quantum computers to solve complex problems exponentially faster than traditional systems.
Traditional computers use bits as the smallest unit of data, which can be either 0 or 1. In contrast, quantum computers use quantum bits, or qubits, which can represent 0, 1, or both 0 and 1 simultaneously due to a phenomenon called superposition. This ability to exist in multiple states at once drastically increases the potential computational power. For instance, while a classical computer processes one solution at a time, a quantum computer can explore many possibilities in parallel, dramatically speeding up calculations for certain types of problems.
Another key principle of quantum computing is entanglement. When qubits become entangled, the state of one qubit is dependent on the state of another, no matter the distance between them. This allows quantum systems to perform highly interconnected calculations more efficiently, leading to faster problem-solving capabilities.
Quantum computing's power comes from its ability to solve problems that are computationally intensive or nearly impossible for classical computers. For example, quantum systems can be used to simulate complex molecular structures, enabling breakthroughs in drug discovery and materials science. In addition, quantum computing has the potential to revolutionize fields like artificial intelligence, optimization, and cryptography by providing vastly superior performance compared to classical methods.
However, building practical quantum computers remains a significant challenge. The qubits used in quantum systems are incredibly sensitive to their environment, and maintaining their stability—known as quantum coherence—is difficult. Current quantum computers require ultra-cold temperatures and other extreme conditions to function effectively, which makes scaling up the technology a complex task.
Despite these challenges, significant progress has been made in the field, with companies like Google Quantum AI and IBM leading the way in quantum computing research. The ultimate goal is to develop a practical, error-corrected quantum computer capable of solving real-world problems at speeds that far surpass the capabilities of classical computers.
In summary, quantum computing represents a paradigm shift in how we approach computation. While it's still in its early stages, its potential to revolutionize industries—from finance and healthcare to transportation and AI—is immense, promising solutions to some of the world’s most pressing challenges.
AI and Machine Learning Powered by Quantum Computing
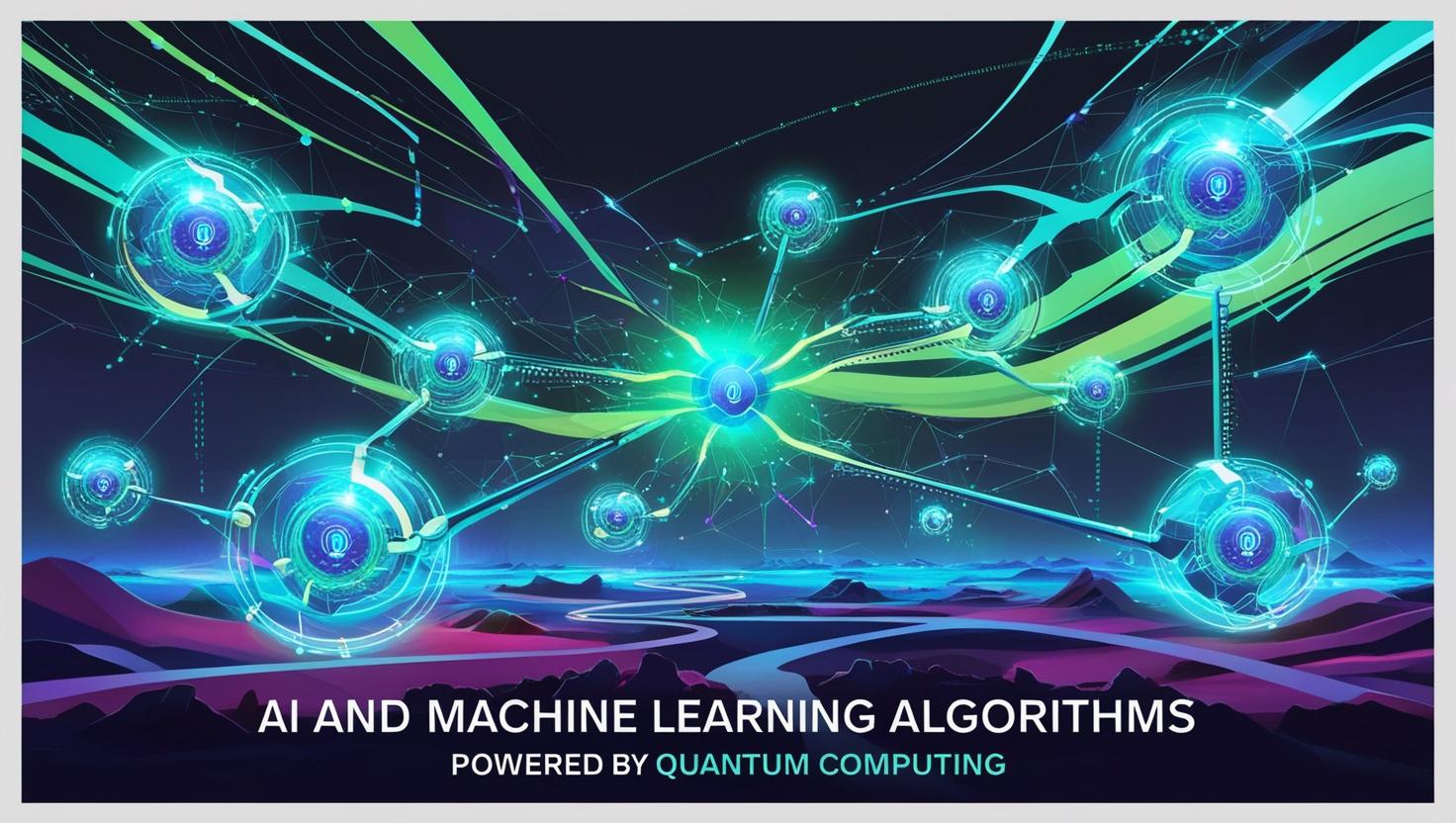
Artificial intelligence (AI) and machine learning (ML) are transforming industries by enabling systems to learn from data, make decisions, and improve over time. However, as the complexity and volume of data grow, traditional classical computing methods struggle to keep up with the computational demands. This is where quantum computing holds immense potential. By leveraging the principles of quantum mechanics, quantum computing can enhance AI and ML capabilities, making it possible to solve problems that were previously out of reach for classical computers.
One of the key advantages of quantum computing in AI and ML is the ability to handle large datasets more efficiently. Quantum computers utilize quantum systems that allow them to process information in parallel through superposition, where qubits can exist in multiple states simultaneously. This enables quantum systems to explore many possible solutions at once, dramatically speeding up tasks like optimization, pattern recognition, and data analysis. For example, a quantum computer can process a large dataset of images or text much faster than a classical computer, enabling more rapid training of AI models.
Another important aspect of quantum computing in AI and ML is quantum entanglement. When qubits become entangled, their states are interconnected, allowing quantum systems to perform complex, multi-dimensional calculations in ways that classical systems cannot. This property has the potential to revolutionize the training of deep learning models. By improving the efficiency of these models, quantum-enhanced AI could unlock new applications in areas like natural language processing, computer vision, and autonomous systems.
Moreover, quantum computing can significantly improve the optimization processes that are central to many machine learning algorithms. Optimization is essential for tasks like tuning the parameters of a neural network or finding the best path for a delivery route. Quantum computers can solve these optimization problems exponentially faster than classical methods, thanks to their ability to evaluate multiple possibilities simultaneously.
One example of quantum computing in AI is Google Quantum AI's work in developing quantum machine learning algorithms. They are exploring how quantum systems can accelerate the training and inference of ML models, especially for tasks that involve large amounts of unstructured data. Quantum computers could also help solve error-corrected models in AI, reducing the limitations posed by noise and errors in data processing.
In summary, quantum computing has the potential to revolutionize AI and machine learning by speeding up data processing, improving model training, and solving complex optimization problems. As quantum technology advances, it could open up new frontiers in AI research, leading to breakthroughs in areas such as personalized medicine, autonomous vehicles, and predictive analytics. The fusion of AI and quantum computing promises to create smarter, more efficient systems that can tackle some of the most complex challenges facing society today.
Quantum Computing in Financial Modeling
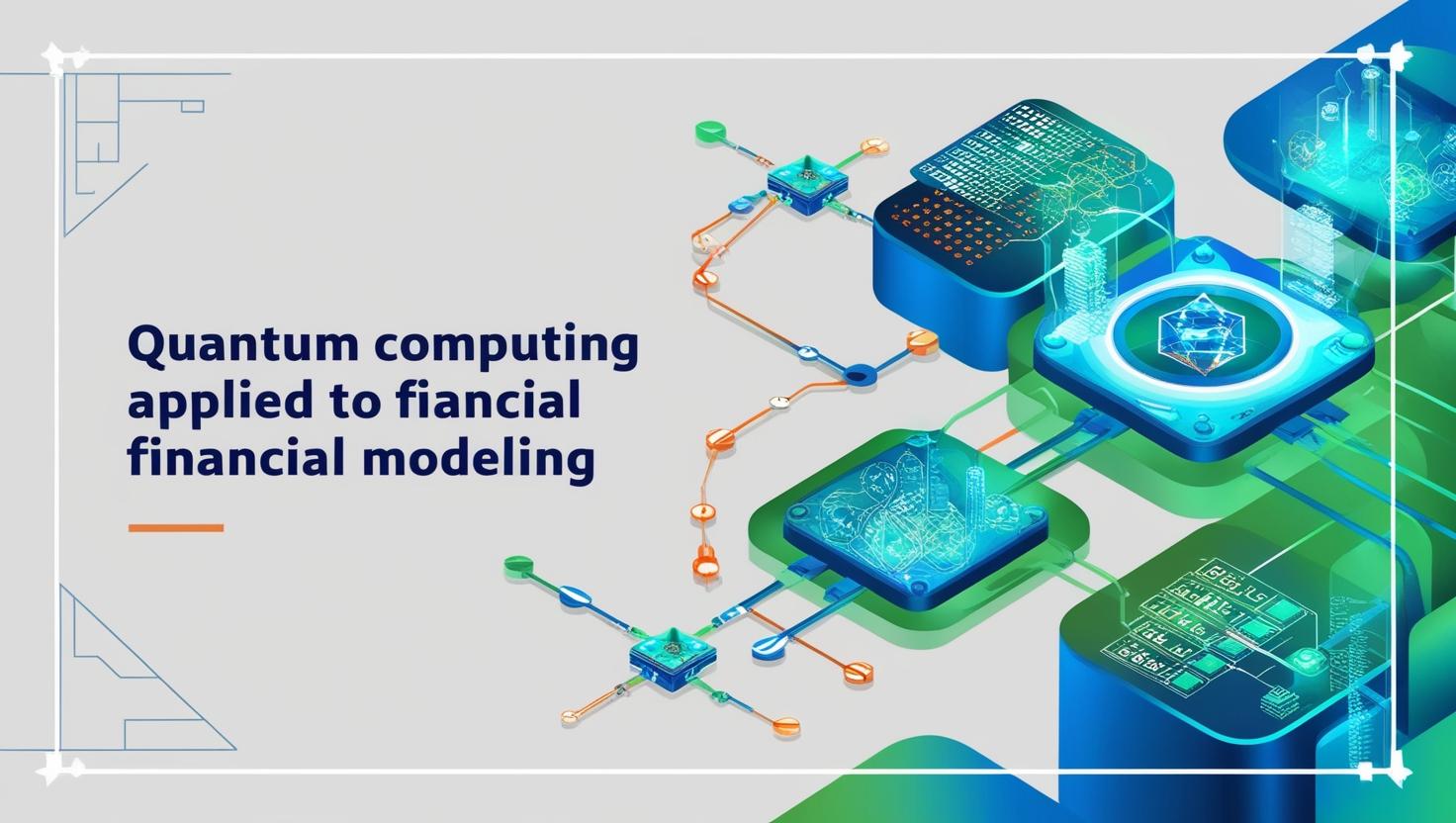
Quantum computing is set to revolutionize financial modeling by providing faster, more efficient solutions for complex problems. Here's a breakdown of how quantum computing is reshaping financial modeling:
1. Risk Analysis and Simulations
- Faster Risk Simulations: Traditional Monte Carlo simulations for risk analysis are time-consuming and require significant computational resources. Quantum computing speeds up these simulations exponentially using quantum systems that process multiple possibilities simultaneously through superposition.
- Real-Time Decision Making: Quantum systems enable real-time risk assessments, allowing financial institutions to make more informed, immediate decisions based on accurate simulations.
- More Accurate Risk Models: By solving complex risk simulations more efficiently, quantum computing provides deeper insights into potential financial risks and forecasts.
2. Portfolio Management Optimization
- Complex Portfolio Optimization: Quantum computing can address the complexity of optimizing portfolios by considering multiple variables simultaneously. This is a highly complex problem for classical computers, especially with an increasing number of assets.
- Quantum Approximate Optimization Algorithm (QAOA): This quantum algorithm can rapidly evaluate thousands of possible portfolio combinations, helping investors to create optimized portfolios with minimal risk and maximum returns.
- Faster Portfolio Strategies: With quantum computing, investors can analyze large sets of data and develop better portfolio strategies in a fraction of the time it takes classical methods.
3. Pricing Complex Financial Derivatives
- Valuation of Derivatives: Quantum computing can assist in pricing financial derivatives (options, futures, swaps) that require intricate mathematical models. Quantum algorithms process a larger number of variables simultaneously to improve accuracy.
- Improved Accuracy: Quantum computing enhances the precision of derivative pricing by considering more complex scenarios, reducing errors in financial predictions.
4. Fraud Detection and Cybersecurity
- Real-Time Fraud Detection: Quantum systems analyze vast datasets quickly, identifying patterns and anomalies that may indicate fraudulent activities or security breaches.
- Enhanced Security: Quantum computing's ability to process data faster and with more accuracy can significantly improve cybersecurity measures in financial institutions.
5. Advancement in Machine Learning and Automated Trading
- Machine Learning Acceleration: Quantum computing enables faster data processing for AI and machine learning algorithms used in finance. This results in more accurate predictive models for customer behavior and financial markets.
- Improved Automated Trading: By analyzing large datasets more efficiently, quantum computing enhances automated trading strategies, providing financial firms with a competitive edge.
6. Future of Quantum Computing in Finance
- Increased Efficiency: As quantum technology matures, it will provide more efficient ways to process financial data, further enhancing financial modeling techniques.
- Transformative Potential: Quantum computing will continue to unlock new possibilities for improving financial strategies, from risk analysis and portfolio optimization to fraud detection and machine learning applications.
In conclusion, quantum computing is transforming financial modeling by enabling faster simulations, better portfolio management, and improved pricing strategies. Its potential to enhance risk analysis, optimize portfolios, and detect fraud makes it a game-changing technology for the finance sector. As quantum computing evolves, it will drive significant advancements in financial modeling and strategy.
Traffic Optimization and Quantum Computing

Traffic congestion is a significant issue for urban areas worldwide, causing delays, increased fuel consumption, and environmental impacts. Traditional methods for traffic optimization typically rely on classical computers to analyze traffic patterns and manage traffic lights. However, as cities grow in size and complexity, these methods struggle to keep up. Quantum computing offers a groundbreaking solution for traffic optimization, providing the computational power needed to address these challenges.
One of the key advantages of quantum computing in traffic management is its ability to solve optimization problems much more efficiently than classical computers. For example, traffic light scheduling is a complex optimization problem that requires considering multiple variables—such as traffic volume, light cycles, and pedestrian crossings—simultaneously. Quantum algorithms can analyze vast amounts of data and provide optimal solutions in real time, minimizing congestion and improving traffic flow.
Quantum systems use superposition and entanglement to evaluate many potential solutions simultaneously. This allows for better prediction and management of traffic patterns, even in highly dynamic environments. By simulating quantum processes, quantum computers can model traffic systems in ways that classical methods cannot, leading to more accurate predictions and real-time adjustments.
Additionally, quantum computing can optimize route planning for logistics and transportation companies. By analyzing traffic data in real time, quantum algorithms can determine the most efficient routes for delivery trucks or public transportation, reducing delays and costs.
In summary, quantum computing holds significant potential for revolutionizing traffic optimization. By leveraging quantum algorithms for real-time data analysis and optimization, cities can reduce congestion, improve traffic flow, and enhance urban mobility. As quantum computing technology advances, its application in traffic management will become more practical, paving the way for smarter, more efficient transportation systems.
Practical Applications of Quantum Computing
Quantum computing is poised to revolutionize various industries by solving complex problems that classical computers cannot efficiently tackle. While still in its early stages, several practical applications of quantum computing are already emerging, particularly in fields such as finance, healthcare, logistics, and materials science.
1. Financial Modeling and Risk Analysis
Quantum computing is ideal for handling complex financial models and risk assessments. Traditional financial modeling relies on Monte Carlo simulations, which are computationally intensive and slow. Quantum algorithms can dramatically speed up these simulations, allowing for real-time risk analysis and more accurate predictions. Quantum computing can also optimize portfolio management by evaluating multiple asset combinations more efficiently than classical methods.
2. Drug Discovery and Healthcare
Quantum computers can simulate molecular interactions with unprecedented accuracy, helping researchers develop new drugs and medical treatments faster. By simulating complex biochemical processes, quantum computing can identify promising drug candidates, speeding up the development of life-saving medications and treatments.
3. Optimization Problems in Logistics
In industries like transportation and supply chain management, optimization is crucial. Quantum computing can solve complex routing and scheduling problems, such as determining the fastest delivery routes or optimizing supply chains, far more efficiently than classical computers. This can result in cost savings, improved delivery times, and better resource allocation.
4. Material Science and Manufacturing
Quantum computing enables researchers to simulate materials at the quantum level, which is particularly useful for designing new materials with unique properties. This has potential applications in industries like electronics, energy storage, and manufacturing, where discovering novel materials can lead to innovations in product design and performance.
5. Artificial Intelligence and Machine Learning
Quantum computing can enhance AI and machine learning by improving data processing speeds and optimization. Quantum algorithms can enable faster training of models, leading to more accurate predictions and insights in fields such as autonomous vehicles, natural language processing, and robotics.
Quantum AI: Google Quantum AI and Other Leading Innovations
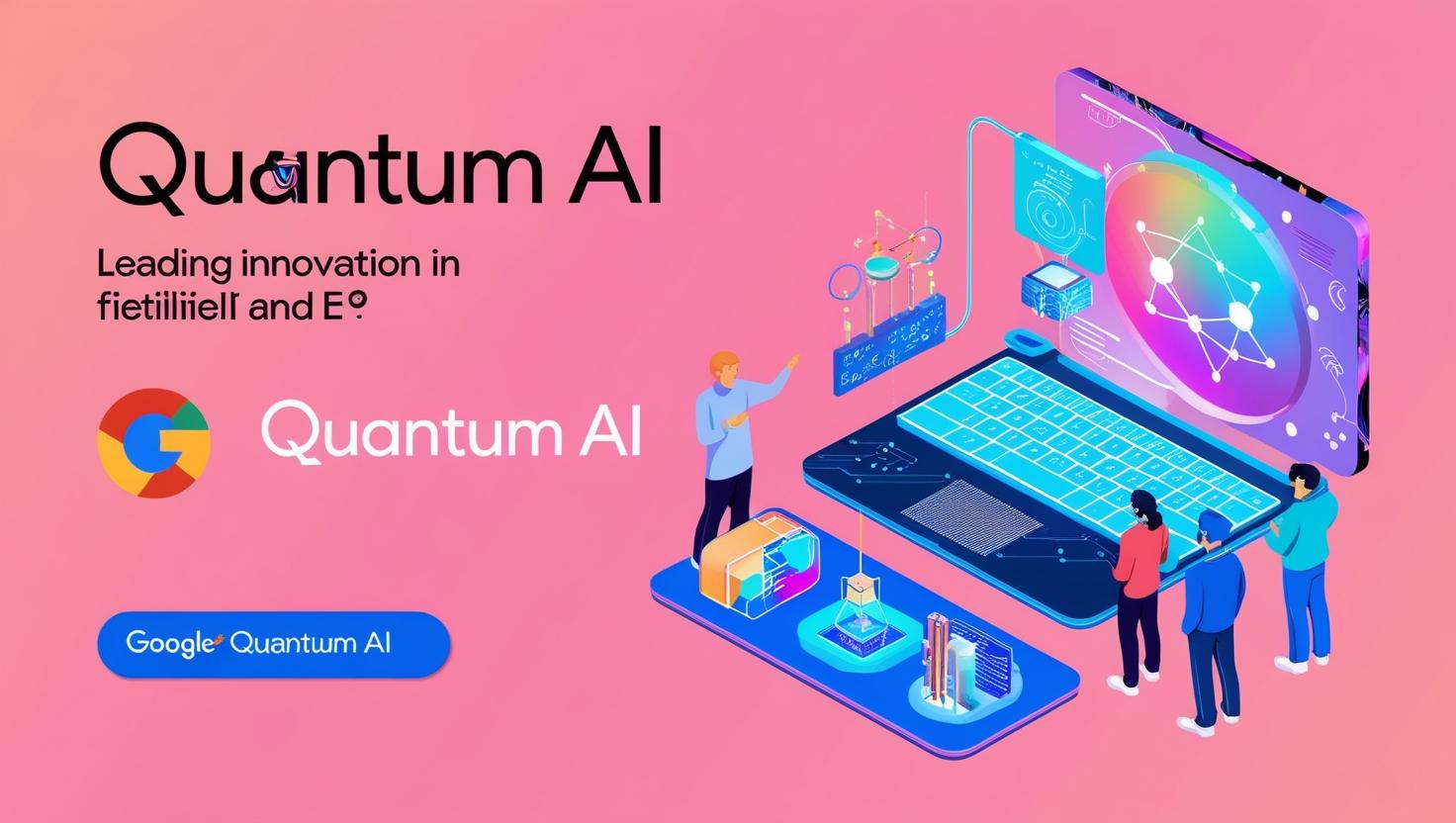
Quantum AI is the fusion of quantum computing and artificial intelligence, combining the power of quantum algorithms with the capabilities of machine learning to solve complex problems that classical computers struggle with. Google Quantum AI is at the forefront of this groundbreaking field, working on innovative quantum solutions to accelerate AI development.
Google’s Quantum AI research focuses on using quantum computing to solve optimization and machine learning problems at unprecedented speeds. Quantum computers utilize quantum systems that can perform many calculations simultaneously through superposition, significantly speeding up tasks like training machine learning models, enhancing data analysis, and solving optimization problems. Google’s quantum advantage in AI lies in its ability to process vast amounts of data in parallel, accelerating AI learning and prediction capabilities.
One key development in this area is Google's work on the quantum neural network. This network leverages quantum computing to improve the speed and efficiency of deep learning models, making AI applications more scalable and effective. Google’s experiments with quantum algorithms like the Quantum Approximate Optimization Algorithm (QAOA) show promise for enhancing AI-driven tasks, such as pattern recognition and predictive analytics.
Beyond Google, several other companies and research institutions are advancing quantum AI. IBM’s Quantum AI research focuses on applying quantum computing to machine learning, enabling more efficient training and optimization of AI models. Microsoft’s Quantum Development Kit also includes tools for building quantum machine learning algorithms.
Furthermore, quantum-enhanced machine learning could revolutionize industries such as healthcare, finance, and logistics. In healthcare, it could lead to more accurate diagnoses by analyzing large datasets, while in finance, it could improve risk modeling and portfolio optimization.
In summary, quantum AI is a rapidly evolving field with the potential to transform industries. With major players like Google Quantum AI leading the charge, quantum computing is poised to unlock new levels of intelligence and problem-solving capabilities across various sectors.
Challenges in Quantum Computing: Classical Computers vs Quantum Systems
Quantum computing promises to revolutionize industries by solving problems that classical computers struggle with, such as complex simulations and optimization tasks. However, as groundbreaking as quantum systems are, there are still significant challenges that need to be addressed before they can fully replace classical computers in real-world applications. Comparing the limitations of classical computers with the potential of quantum systems helps shed light on the hurdles that quantum computing faces.
1. Error Rates and Stability of Quantum Systems
One of the most significant challenges in quantum computing is maintaining stability and minimizing error rates. Quantum systems are extremely sensitive to external disturbances, such as temperature fluctuations or electromagnetic interference. This sensitivity makes error correction one of the most pressing issues. Unlike classical computers, where data is stored in binary form (1s and 0s), quantum computers use quantum bits (qubits) that can exist in multiple states simultaneously. The process of maintaining the quantum state and reducing the error rates is extremely difficult. Error-corrected quantum computing is still an area of intense research, with methods like the surface code showing potential, but no definitive solution has yet been established.
2. Quantum Decoherence
Quantum decoherence occurs when a qubit loses its quantum state due to interaction with the environment. This problem makes it difficult to maintain the delicate quantum states needed for accurate computation. Classical computers, on the other hand, do not suffer from this issue as their bits are not as sensitive to environmental changes. Researchers are actively working on improving the stability of quantum systems, but overcoming decoherence remains a major hurdle for large-scale quantum computing.
3. Scalability of Quantum Hardware
Another key challenge is the scalability of quantum hardware. Current quantum processors have only a limited number of qubits. To achieve practical real-world applications of quantum computing, such as simulating complex molecules or optimizing large-scale logistical problems, quantum systems need to scale to thousands or even millions of qubits. Quantum hardware improvements, such as superconducting qubits and trapped ion qubits, are being explored, but building scalable quantum computers that can outperform classical systems remains a significant challenge.
4. Algorithm Development
While classical computers use well-established algorithms, quantum computing requires entirely new quantum algorithms that take advantage of quantum mechanics. The complexity of developing and optimizing quantum algorithms for tasks like machine learning, cryptography, and optimization makes it a challenging field of study. Quantum algorithms such as Shor’s algorithm and Grover’s algorithm show the promise of quantum speedups, but practical real-time applications are still limited. As the field evolves, more efficient and practical algorithms must be developed for specific industries.
5. Integration with Classical Systems
In most practical scenarios, quantum computing will not fully replace classical computers but will work alongside them to solve specific problems that are computationally expensive for classical systems. Quantum computing research suggests that hybrid models—combining classical and quantum methods—are the most viable approach for the near future. However, seamless quantum integration into existing infrastructure poses its own set of challenges, especially in areas like cloud computing, where businesses need to incorporate quantum capabilities without overhauling their entire technology stack.
6. Cost and Accessibility
Building and maintaining quantum systems is extremely expensive, requiring advanced quantum hardware and special environments to isolate qubits. The infrastructure needed for quantum computing, such as cryogenic systems to cool the qubits, adds to the cost. For quantum computing to become widely accessible, innovations are needed to reduce these costs, making quantum computing more affordable and scalable for both research and commercial applications.
7. Applications of Quantum Computing
Despite the challenges, the potential applications of quantum computing are immense. In fields like AI and machine learning, quantum systems could provide faster data processing, leading to better optimization, traffic optimization, and predictive analytics. Quantum systems have also shown promise in financial modeling, where they can optimize portfolios and simulate complex financial scenarios. In healthcare, quantum mechanical simulations could accelerate drug discovery, and in materials science, quantum computing could help develop new materials with unique properties.
Future of Quantum Computing: What’s Next?
Quantum computing is still in its early stages, but its potential to transform industries and solve previously intractable problems is immense. As research and development continue to advance, the future of quantum computing promises breakthroughs that will impact a variety of sectors, from finance to healthcare, logistics, and beyond.
1. Error Correction and Stability Improvements
One of the key areas of focus in the coming years will be improving the stability and error correction of quantum systems. Currently, quantum computers are prone to errors due to quantum decoherence and noise. Overcoming these issues with error-corrected quantum systems will be essential for scaling quantum computers and making them reliable for real-world applications. Advancements in quantum error correction codes and hardware improvements are expected to play a significant role in this area.
2. Scaling Quantum Systems
The next step for quantum computing is scaling up the number of qubits while maintaining their coherence. Progress in quantum hardware, including advancements in quantum hardware such as superconducting qubits and trapped ions, will allow for more powerful quantum systems. Achieving the necessary qubit count and connectivity to perform large-scale quantum computations will open up new possibilities for tackling complex problems like drug discovery, optimization, and machine learning.
3. Quantum Software and Algorithms
Along with hardware improvements, there will be a focus on developing new quantum algorithms tailored for specific industries and applications. Quantum computing will likely be used in tandem with classical computing to form hybrid systems that leverage the strengths of both. As quantum algorithms improve, we can expect practical applications in areas like AI and machine learning, quantum-enhanced cryptography, and optimization problems.
4. Industry Adoption and Quantum Cloud
As quantum systems mature, the integration of quantum computing with existing infrastructure will be crucial. The rise of quantum cloud services will allow businesses to access quantum capabilities without the need for expensive hardware investments. This will drive broader adoption across industries, enabling companies to explore quantum solutions for complex problems.
Conclusion
The future of quantum computing is both promising and challenging, with the potential to reshape industries and solve complex problems that are beyond the reach of classical computers. As advancements in quantum hardware, error correction, and quantum algorithms continue, the gap between theoretical possibilities and real-world applications is narrowing. The next few years will be crucial in determining how quickly quantum systems can scale and become reliable for practical use.
While the journey towards fully realizing the power of quantum computing involves overcoming significant hurdles such as stability and error rates, the progress made so far is nothing short of revolutionary. From enhancing AI and machine learning to optimizing financial models and advancing drug discovery, quantum computing will have a transformative impact across multiple sectors. As quantum cloud services expand and quantum systems become more accessible, businesses and researchers will be able to leverage this powerful technology without the need for specialized infrastructure.
In conclusion, quantum computing represents a new frontier in technology. Although it is still in its early stages, its potential is vast, and continued research will unlock new capabilities that could change the way we approach problem-solving, optimization, and data analysis. The future of quantum computing holds the promise of innovations that can accelerate progress in ways we can only begin to imagine today.